DepMap | Models
Cell models and linked datasets
Approximately 1,600 human cancer cell lines are available to scientists worldwide and these are a useful resource for cancer research and drug development. Over the past decade the Sanger Institute has played a central role in the genetic and functional analysis of these cancer cell lines. As we enter the era of precision cancer medicine our current cell model collection limits our ability to treat more patients in a molecularly defined manner due to poor representation of some cancer types, insufficient numbers to capture the genetic diversity of cancer, lack of clinical outcome data and lack of comparison to normal reference samples.
We aim to generate a new comprehensive and diverse collection of highly-annotated cancer cell models. This will include derivation and genomic characterisation of new patient-derived 3D organoid cultures, 2D cell lines and the generation of genetically-engineered isogenic cancer models. Cell model passports provide a gateway to access all relevant information about our cell models.D
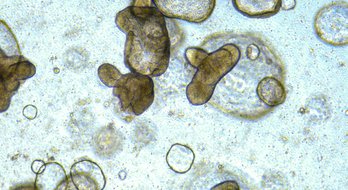
The cell model passports provides a single location where information on Sanger DepMap cell models is available in a user-friendly environment.
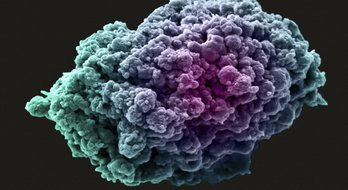
Together with CRUK, we aim to derive approximately 1000 new cancer cell models, annotated with genomic and clinical data as part of the HCMI.
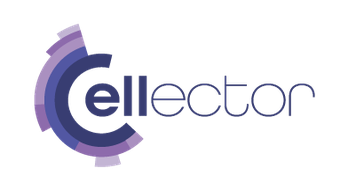
A tool that allows researchers to select the most relevant cancer cell lines in a genomic-guided fashion, based on graph theory and market basket analysis.
DepMap | Drugs
Drug sensitivity and molecular biomarkers
Targeted molecular therapeutics are increasingly used for the treatment of cancer patients. These strategies are based on our understanding of the genes that are causally implicated in cancer and clinical observations that molecular alterations in cancer genomes strongly influence patient response to drugs. Many cancer drugs currently used in the clinic or in clinical development have not been linked with specific genetic events that may specify their optimal therapeutic effectiveness.
We are performing large-scale differential drug sensitivity screens in cancer cell models and incorporating detailed genomic analyses to systematically identify drug response biomarkers. This includes both single agent and drug combinations screens. Large numbers of cancer cell models are required to encompass the heterogeneity of human cancer to discover novel efficacious therapeutic strategies in defined patient populations. This information can be used to inform the optimal clinical application of cancer drugs, as well as having significant effects on the design, cost and ultimate success of new cancer drug development.
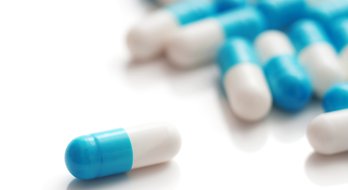
The Genomics of Drug Sensitivity in Cancer (GDSC) project aims to identify the molecular features of cancers that predict response to anti-cancer drugs.
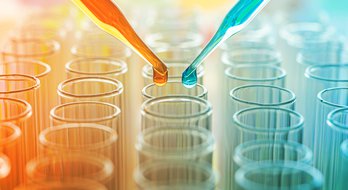
We are undertaking a systematic, large-scale effort to define the activity of drug combinations across a collection of cancer genomes as a strategy to improve patient responses and prevent the emergence of drug resistance.
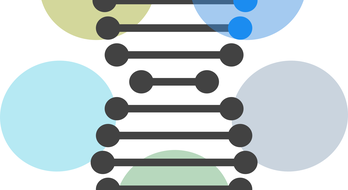
DoRothEA is a tool to search for transcription factor-drug interactions in cancer, where TFs are considered sensors of pathways and alternative markers
DepMap | Genes
Genetic screens to identify cancer dependencies
The complexity and diversity of cancer genomes represents a significant challenge when developing new cancer therapies. Specifically, identifying cellular signalling pathways and processes whose perturbation selectively kills cancer cells while sparing normal cells remains acutely difficult. This is because our understanding of which proteins are necessary for cancer cell survival is incomplete. Furthermore, our understanding of cellular networks and processes is relatively poor in normal cells, let alone in the context of cancer cells with their myriad of molecular alterations. Systematic and unbiased approaches to identify critical dependencies in cancer cells could significantly expand the repertoire of new drug targets for future development.
Genome-editing technologies such as CRISPR-Cas9 are a powerful tool for studying gene function in normal and diseased cells. This approach takes advantages of the programmable nature of single guide RNAs (sgRNAs) to perform genome-wide functional genetic screens across a diverse array of cellular models and systems. We are using CRISPR-Cas9 synthetic-lethal genetic screens to map gene function and identify dependencies across a diverse collection of cancer cell models.
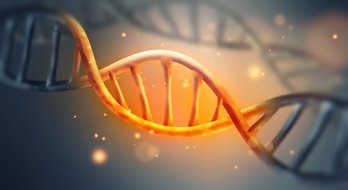
This project aims to use CRISPR-Cas9 editing technology across a large number of cancer cell models at a genome-wide level to switch off each gene individually to determine cell specific dependencies.
DepMap | Analytics
Software and tools for analysing DepMap data
The development of a Cancer Dependency Map will require the development of novel computational algorithms for the processing and analysis of new datasets. Furthermore, to fully exploit our large-scale multi-dimensional genomic and functional datasets we will require completely novel approaches for data integration and visualization beyond what is currently available. We are developing new tools and software to support the creation of a DepMap and these will be made available to the research community.
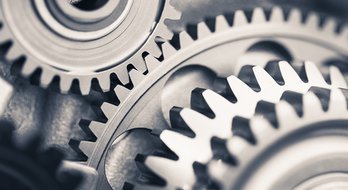
Analyse drug sensitivity data with gdscIC50, visualise dose-responses with gdscDataViewR and perform biomarker analysis using GDSCtools.
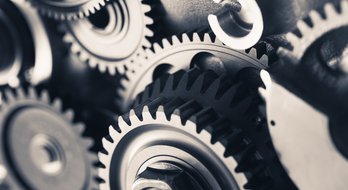
An R package for unsupervised identification and correction of gene independent cell responses to CRISPR-cas9 targeting
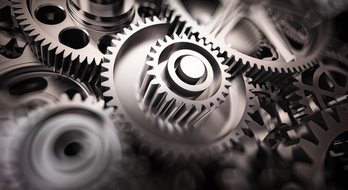
A Bayesian method that jointly analyses screens performed with the same guide RNA library to improve hit identification, reducing sample requirement by 2.5-fold.
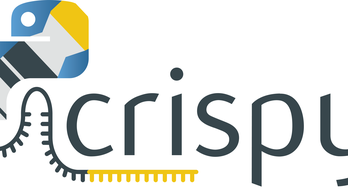
Supervised and single-sample copy-number correction tool for CRISPR-Cas9 screens
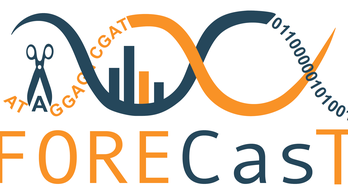
An online tool for the computational prediction of editing outcomes resulting from Cas9-induced double strand breaks.
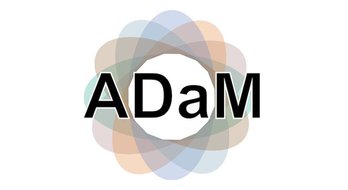
ADaM (Adaptive Daisy Model): an R package for discriminating between core fitness and context-specific fitness genes in large-scale gene essentiality datasets.